My impressions after a little over a month of using the AI-assisted IDE Cursor full-time
A Month(ish) of Vibes with Cursor
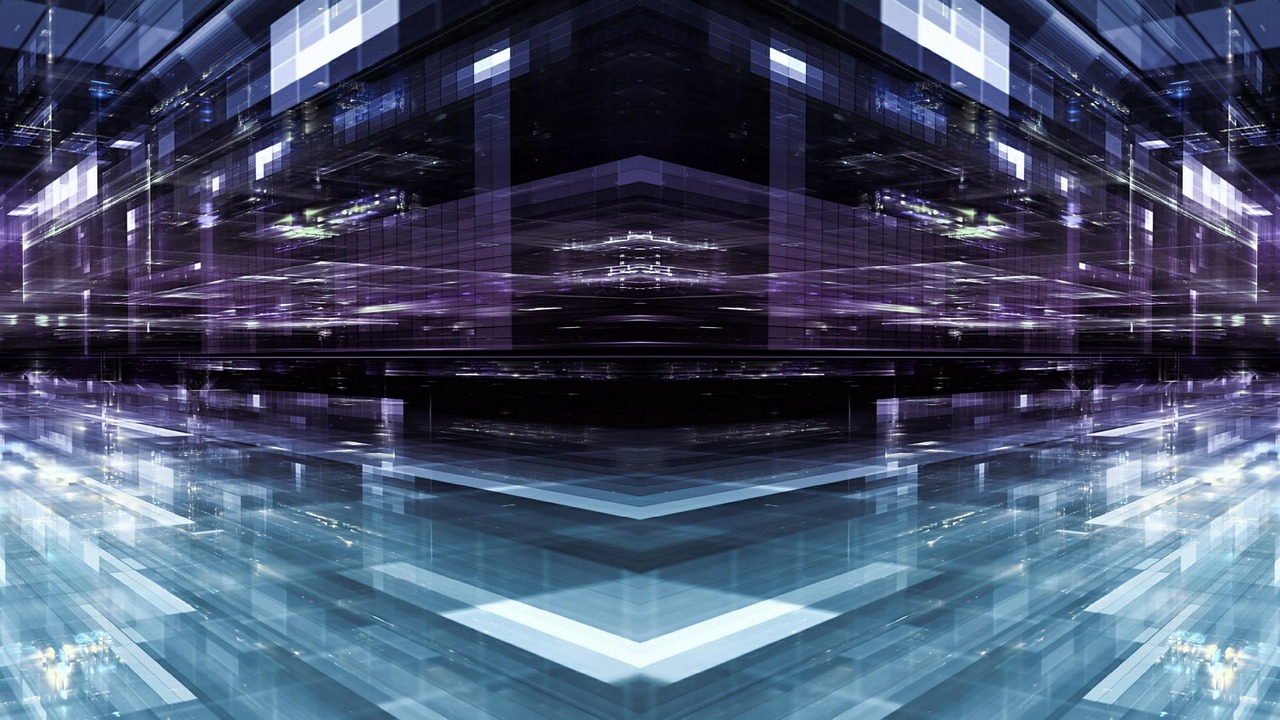
My impressions after a little over a month of using the AI-assisted IDE Cursor full-time
Good evening from Greece! While I’m catching up on the Australian Grand Prix, I wanted to share this great thread that analogizes post-training optimizations in LLMs to in-season car improvements in Formula 1:
Consider F1, most of the teams show up to the beginning of the year with a new chassis and engine. Then, they spend all year on aerodynamics and systems changes (a minor over simplification), and can dramatically improve the performance of the car. The best F1 teams improve way more during a season.
I’m a huge fan of the ATProtocol, the protocol that powers Bluesky, and not just for the protocol itself. The community of builders that has grown around it (the Atmosphere) is impressive, and we are starting to see some really interesting projects reach escape velocity.
Tangled.sh is one of those, allowing users to host git repositories on lightweight headless servers called “knots,” while consolidating all knots on the network into a single view via the Tangled AppView. Exciting days ahead for decentralization!
Inspired by Carlos Vargas, who was in turn inspired by Simon Willison, I’ve decided to start doing link posts here. Short-form commentary is important, as is sharing links, and I don’t want that to be the sole domain of ephemeral, high-volume social media. Maybe it’ll end up good newsletter fodder for those who prefer that format.
via Carlos Vargas on Bluesky; follow Simon Willison on Bluesky too.
Reflecting back on a tumultuous 2024, I decide that there is only one way to describe the year, the events, and the people who showed up.
Returning to Greece for the first time, I find a deeper connection to my family and culture than ever before
A tutorial for receiving APRS traffic with a software-defined radio and forwarding it to the Internet
A how-to for linking an AWS API Gateway REST API to a Step Function using Amazon’s CDK
A short ode to and remembrance of my Yiayia, who’s story is like that of many other Greeks who fled war and famine during the Greek Civil War but who’s impact far exceeded her tiny frame.
RoamLab is a knowledge management framework for makers and thinkers. This post outlines the RoamLab.
← previous | This site is part of the Data People Writing Stuff webring random | index | what is this? |
next → |